AI for humans.
Safe. Reliable. Empowering.
Wir bei QualityMinds bauen qualitativ hochwertige KI-Lösungen, die genau auf die Bedürfnisse unserer Kunden zugeschnitten sind. Wir zeichnen uns aus durch Präzision und Klarheit. Wir beraten und entwickeln umfassend und ganzheitlich - von der Ideengenerierung und dem ersten Entwurf über die Grundkonzeption, deren Umsetzung bis hin zur operativen Unterstützung.
Showcases
Im QualityMinds AI LAB veröffentlichen wir verschiedene KI Showcases, die aus unseren Forschungs- und Kundenprojekten stammen.

Explorative Datenanalyse für KI-Testing
Bei der Qualitätssicherung von KI, insbesondere im Bereich des autonomen Fahrens, helfen interaktive Visualisierungen dabei, Schwachstellen der KI, wie die Erkennung von Fußgängern, zu identifizieren. Das vorgestellte Tool integriert sich nahtlos in Jupyter-Umgebungen und erleichtert die explorative Datenanalyse ohne großen Mehraufwand.

Bewegungsvorhersage mit KI-Technologie
Das Forschungsprojekt “KI-ATTENTION” untersucht die Vorhersage von Verletzungen in Echtzeit mithilfe von Künstlicher Intelligenz. In diesem Rahmen wurde ein neues Modell (CIST-GCN) entwickelt, das durch eine interpretierbare Spatio-Temporale Graph Convolutional Network-Technologie eine präzisere Vorhersage menschlicher Bewegungen ermöglicht.
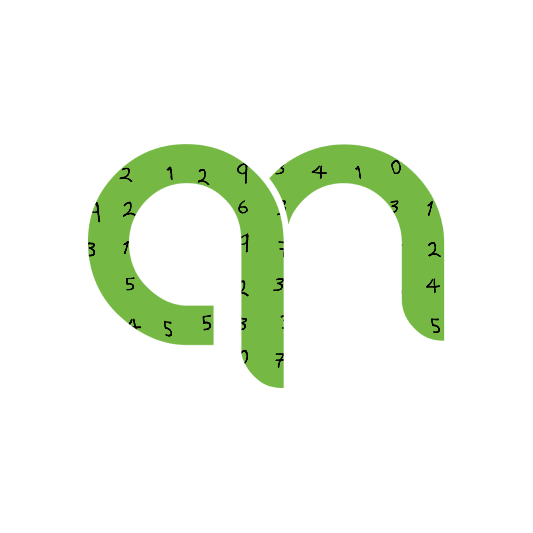
Interaktive Visualisierung: KI-Schwächen erkennen
Eine KI, die handgeschriebene Zahlen erkennt, ist eine typische Einstiegsübung im Machine Learning, und ein Modell aus diesem Showcase erreicht eine Genauigkeit von 99,2%. Doch selbst kleine Änderungen wie Verschiebungen der Eingabe können das Modell leicht ins Stolpern bringen, was auf die “Sprödigkeit” von KI hinweist – ein häufiges Phänomen, das durch spezielle Teststrategien und robustes Training adressiert werden kann.

Präzise 3D-Rekonstruktion durch Gaussian Splatting
3D Gaussian Splatting ist eine Technik zur Darstellung von 3D-Daten durch die Verwendung von Gauss’schen Verteilungen, um komplexe Formen effizient zu modellieren und zu analysieren. Der Trainingsprozess umfasst die Anpassung der Gauss’schen Parameter durch Optimierung, um 3D-Objekte präzise zu rekonstruieren und in Bereichen wie Robotik, medizinischer Bildgebung und virtueller Realität anzuwenden.
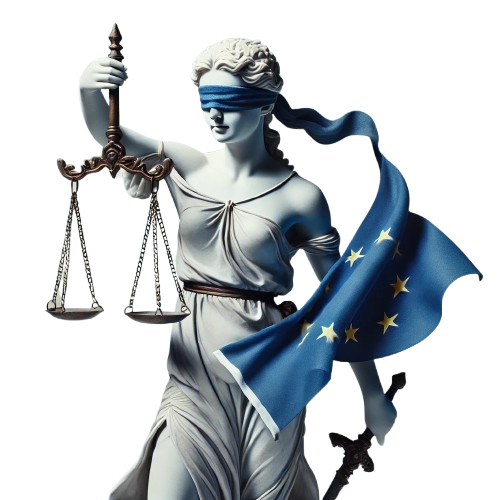
EU AI Act Chatbot
Unser Chatbot bietet schnelle und präzise Antworten auf Fragen zum EU AI Act, ohne dass Du dich durch lange Dokumente arbeiten musst. Er nutzt die fortschrittliche GraphRAG-Technologie, um relevante Informationen aus einem Wissensgraphen gezielt abzurufen und so die Suche zu erleichtern.
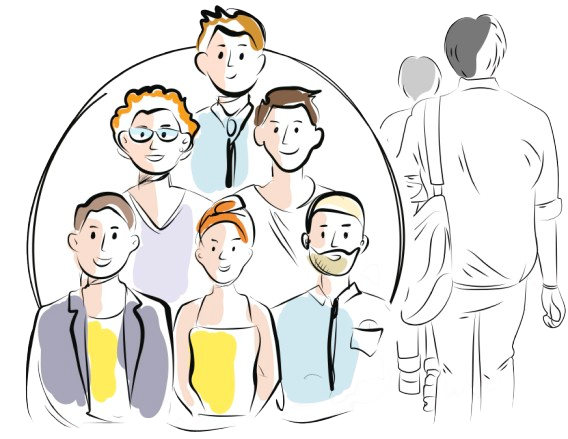
KI-gestützter Wissenstransfer
In den nächsten Jahren stehen deutsche Unternehmen vor einem enormen Verlust an Fachkräften durch Pensionierungen, was den Abfluss von wertvollem Wissen zur Folge haben könnte. QualityMinds hat ein mehrschichtiges Konzept entwickelt, das durch gezielte Maßnahmen das Know-how sichert und Unternehmen dabei unterstützt, gestärkt aus dieser Transformation hervorzugehen.
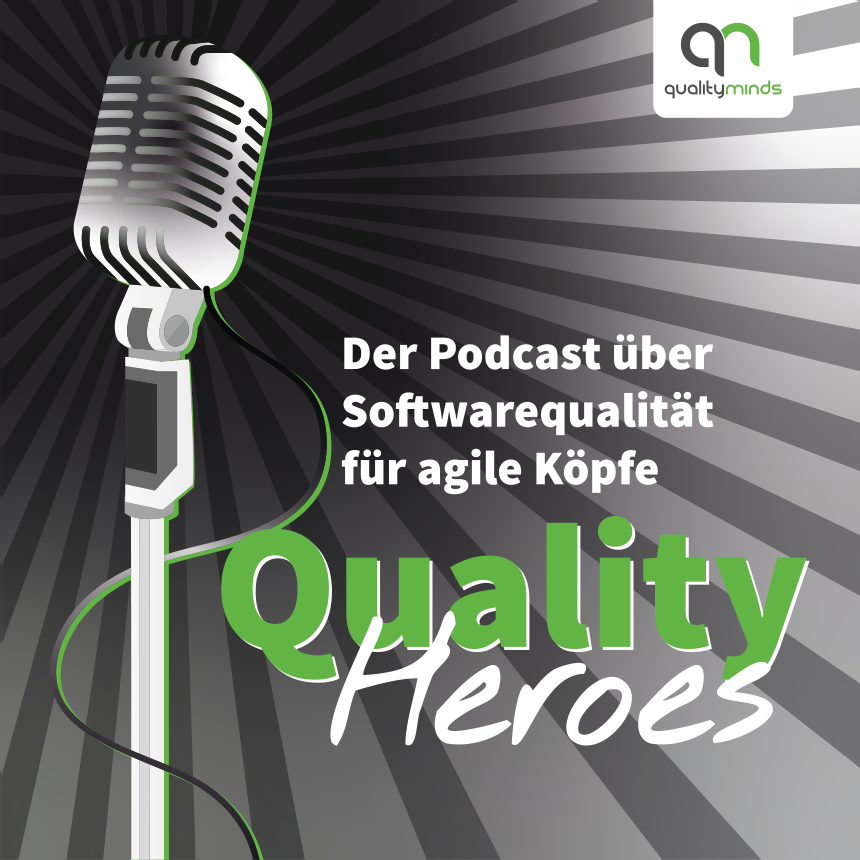
Podcast: Was ist das AI LAB?
In Episode 42 von QualityHeroes werfen wir gemeinsam mit Eva, Niels und Julian einen ersten Blick auf unser neuestes KI-Projekt! Erfahrt, wie wir Künstliche Intelligenz in neue Bahnen lenken und welche Herausforderungen uns dabei begegnet sind. Seid gespannt auf Einblicke, die zeigen, wie KI schon bald unseren Alltag prägen könnte – viel Spaß beim Zuhören!
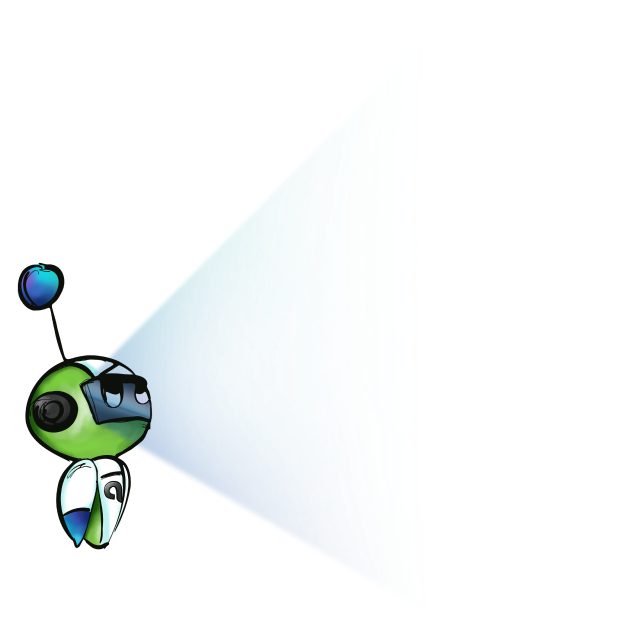
Wir haben Antworten.
Professionelle KI-Services für dein Unternehmen.
Wir beraten unsere Kunden, bieten Workshops an und arbeiten praxisnah an Forschungs- und IT-Projekten.
Ob Automobilindustrie, E-Commerce, öffentlicher Sektor oder Medien & Telekommunikation – unsere Expert:innen haben bereits Projekte in und mit ganz unterschiedlichen Branchen entwickelt, vorangetrieben und realisiert. Unser Know-how geben wir gerne weiter, auch an dein Unternehmen.
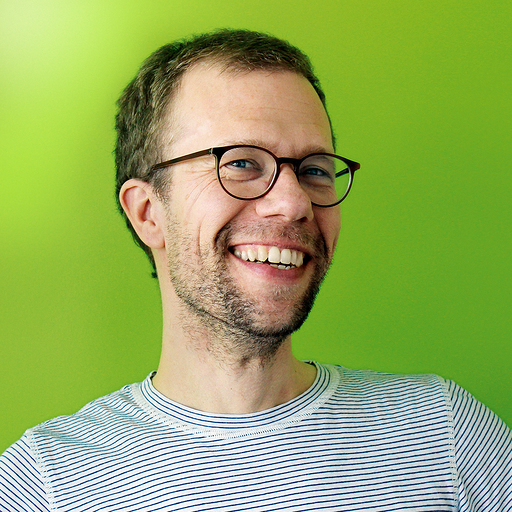
Professionelle KI-Services für dein Unternehmen.
Wir beraten unsere Kunden, bieten Workshops an und arbeiten praxisnah an Forschungs- und IT-Projekten.
Ob Automobilindustrie, E-Commerce, öffentlicher Sektor oder Medien & Telekommunikation – unsere Expert:innen haben bereits Projekte in und mit ganz unterschiedlichen Branchen entwickelt, vorangetrieben und realisiert. Unser Know-how geben wir gerne weiter, auch an dein Unternehmen.
Research Papers & Talks
Unser Wissen fundiert auf jahrelanger Forschung in Bereichen wie Autonomes Fahren, Sicherheit von Machine Learning oder Personalisiertes Lernen.
Data Bias According to Bipol: Men are Naturally Right and It is the Role of Women to Follow Their Lead
Abstract
We introduce new large labeled datasets on bias in 3 languages and show in experiments that bias exists in all 10 datasets of 5 languages evaluated, including benchmark datasets on the English GLUE/SuperGLUE leaderboards. The 3 new languages give a total of almost 6 million labeled samples and we benchmark on these datasets using SotA multilingual pretrained models: mT5 and mBERT. The challenge of social bias, based on prejudice, is ubiquitous, as recent events with AI and large language models (LLMs) have shown. Motivated by this challenge, we set out to estimate bias in multiple datasets. We compare some recent bias metrics and use bipol, which has explainability in the metric. We also confirm the unverified assumption that bias exists in toxic comments by randomly sampling 200 samples from a toxic dataset population using the confidence level of 95% and error margin of 7%. Thirty gold samples were randomly distributed in the 200 samples to secure the quality of the annotation. Our findings confirm that many of the datasets have male bias (prejudice against women), besides other types of bias. We publicly release our new datasets, lexica, models, and codes.
Fairness and Bias in Multimodal AI: A Survey
Abstract
The importance of addressing fairness and bias in artificial intelligence (AI) systems cannot be over-emphasized. Mainstream media has been awashed with news of incidents around stereotypes and other types of bias in many of these systems in recent years. In this survey, we fill a gap with regards to the relatively minimal study of fairness and bias in Large Multimodal Models (LMMs) compared to Large Language Models (LLMs), providing 50 examples of datasets and models related to both types of AI along with the challenges of bias affecting them. We discuss the less-mentioned category of mitigating bias, preprocessing (with particular attention on the first part of it, which we call preuse). The method is less-mentioned compared to the two well-known ones in the literature: intrinsic and extrinsic mitigation methods. We critically discuss the various ways researchers are addressing these challenges. Our method involved two slightly different search queries on two reputable search engines, Google Scholar and Web of Science (WoS), which revealed that for the queries 'Fairness and bias in Large Multimodal Models' and 'Fairness and bias in Large Language Models', 33,400 and 538,000 links are the initial results, respectively, for Scholar while 4 and 50 links are the initial results, respectively, for WoS. For reproducibility and verification, we provide links to the search results and the citations to all the final reviewed papers. We believe this work contributes to filling this gap and providing insight to researchers and other stakeholders on ways to address the challenges of fairness and bias in multimodal and language AI.
Context-based Interpretable Spatio-Temporal Graph Convolutional Network for Human Motion Forecasting
Abstract
Human motion prediction is still an open problem extremely important for autonomous driving and safety applications. Due to the complex spatiotemporal relation of motion sequences, this remains a challenging problem not only for movement prediction but also to perform a preliminary interpretation of the joint connections. In this work, we present a Context-based Interpretable Spatio-Temporal Graph Convolutional Network (CIST-GCN), as an efficient 3D human pose forecasting model based on GCNs that encompasses specific layers, aiding model interpretability and providing information that might be useful when analyzing motion distribution and body behavior. Our architecture extracts meaningful information from pose sequences, aggregates displacements and accelerations into the input model, and finally predicts the output displacements. Extensive experiments on Human 3.6M, AMASS, 3DPW, and ExPI datasets demonstrate that CIST-GCN outperforms previous methods in human motion prediction and robustness. Since the idea of enhancing interpretability for motion prediction has its merits, we showcase experiments towards it and provide preliminary evaluations of such insights here.
Fooling Neural Networks for Motion Forecasting via Adversarial Attacks
Abstract
Human motion prediction is still an open problem, which is extremely important for autonomous driving and safety applications. Although there are great advances in this area, the widely studied topic of adversarial attacks has not been applied to multi-regression models such as GCNs and MLP-based architectures in human motion prediction. This work intends to reduce this gap using extensive quantitative and qualitative experiments in state-of-the-art architectures similar to the initial stages of adversarial attacks in image classification.The results suggest that models are susceptible to attacks even on low levels of perturbation. We also show experiments with 3D transformations that affect the model performance, in particular, we show that most models are sensitive to simple rotations and translations which do not alter joint distances. We conclude that similar to earlier CNN models, motion forecasting tasks are susceptible to small perturbations and simple 3D transformations.
Using AI and Generative AI for Personalized Learning
Abstract
Can AI and Generative AI improve knowledge work, knowledge management, and learning in organizations? In this talk, we will emphasize that the applications for knowledge workers, many executives, and L&D (Learning & Development) professionals are indeed enormous. Based on concrete use cases and applications that we have developed within QualityMinds GmbH, we will illustrate how future solutions could look like, e.g. privacy compliant information processing and knowledge transfer according to the EU AI Act, while using the AI and Generative AI capabilities of LLMs (Large Language Models) and providing customized learning materials by using a Smart Content Crawler. On the one hand, our talk will highlight the technical aspects of using and fine-tuning LLMs, combined with a small demonstration, and on the other hand, we will discuss the challenges (e.g. the urgent need for knowledge transfer in the face of retirement waves and the increasing individualization of learning needs) that organizations face today and in the coming years. It is only through a balanced combination of cultural and technical aspects that the potential of AI for learning and knowledge in organizations can really unfold.
WSAM: Visual Explanations from Style Augmentation as Adversarial Attacker and Their Influence in Image Classification
Abstract
Currently, style augmentation is capturing attention due to convolutional neural networks (CNN) being strongly biased toward recognizing textures rather than shapes. Most existing styling methods either performa low-fidelity style transfer or a weak style representation in the embedding vector. This paper outlines a style augmentation algorithm using stochastic-based sampling with noise addition to improving randomization on a general linear transformation for style transfer. With our augmentation strategy, all models not only present incredible robustness against image stylizing but also outperform all previous methods and surpass the state of-the-art performance for the STL-10 dataset. In addition, we present an analysis of the model interpretations under different style variations. At the same time, we compare comprehensive experiments demonstrating the performance when applied to deep neural architectures in training settings.
Highly Automated Corner Cases Extraction: Using Gradient Boost Quantile Regression for AI Quality Assurance
Abstract
This work introduces a method for Quality Assurance of Artificial Intelligence (AI) Systems, which identifies and characterizes “corner cases”. Here, corner cases are intuitively defined as “inputs yielding an unexpectedly bad AI performance”. While relying on automated methods for corner case selection, the method relies also on human work. Specifically, the method structures the work of data scientists in an iterative process which formalizes the expectations towards an AI under test. The method is applied in a use case in Autonomous Driving, and validation experiments, which point at a general effectiveness of the method, are reported on. Besides allowing insights on the AI under test, the method seems to be particularly suited to structure a constructive critique of the quality of a test dataset. As this work reports on a first application of the method, a special focus lies on limitations and possible extensions of the method.